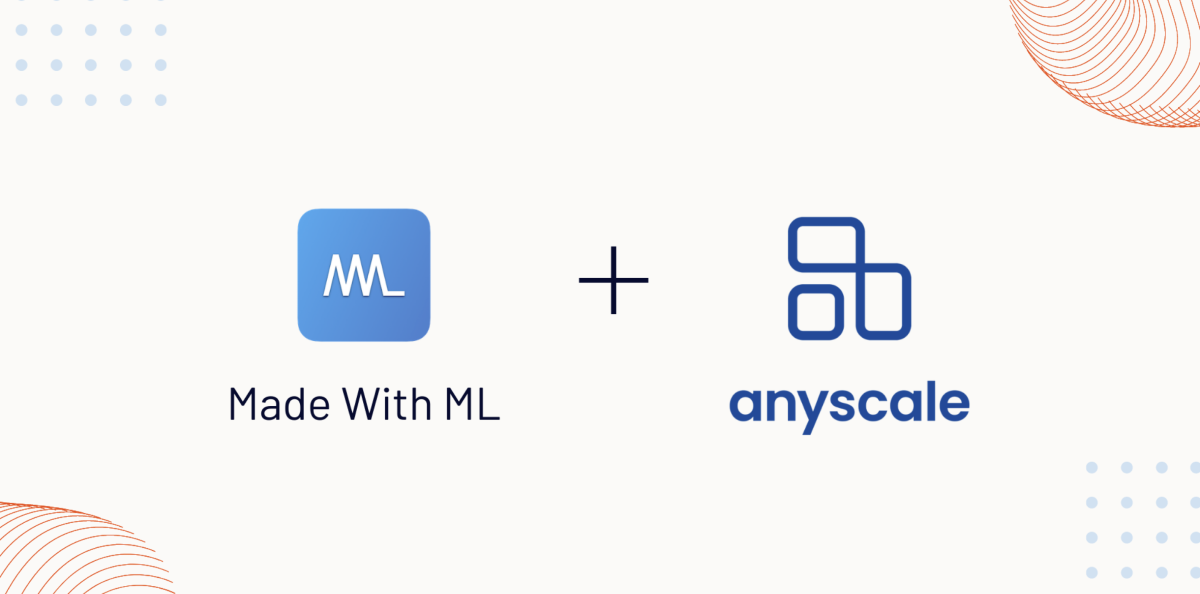
I’m excited to share that Made With ML and I are now part of the Anyscale team! Check out our new ML for Developers course where we make it even easier to develop and productionize ML – based on learnings from 8+ years of helping machine learning teams get to production.
Anyscale is the team behind Ray — a framework for scaling and productionizing AI applications, especially LLMs and generative AI. Ray consists of a core distributed runtime along with libraries for scaling ML workloads and is widely adopted by companies like OpenAI, Spotify, Netflix, Instacart, Doordash + many more. |
Over the past several years through Made With ML, I’ve had the opportunity to help dozens of F500 companies and startups build out their ML platforms and launch high-impact ML applications on top of them. My main objective was to help teams accelerate the maturity of their ML workflows. For early teams, this involved transitioning from manually training models and ad-hoc serving to a mature state with MLOps integrations and CI/CD for training deployment. And for more mature teams, this involved slowly improving on rigid solutions (Kubeflow, SageMaker, Spark ML, Vertex, etc.) to be more scalable, open (ML integrations), and developer-friendly workflows.
It was at the same time, I started Made With ML to share best practices for delivering value with ML in production. The platform gained organic community traction beyond my expectations, rapidly becoming one of the most popular ML GitHub repositories with 40K+ community members, 1M+ monthly visitors across industry and academia.
While this was an amazing experience, it was also a humbling one because there were several recurring obstacles that all teams (regardless of context) encountered that I didn’t have great solutions for. With our new ML for Developers course, we directly address all of these challenges.
Scale: Whether it was the data size, model size, or serving volume, one of these would eventually hit a bottleneck. This was a problem when I first started Made With ML, but it’s far more pervasive today with the explosion of LLMs and generative AI workloads.
Integrations: It was extremely difficult to create an end-to-end machine learning system without a foundation that could connect different ML workloads (ingesting data, training, tuning, serving) and integrate with MLOps components (tracking, testing, monitoring, CI/CD). This often involved using a collection of specialized systems and painfully stitching together all the required pieces. At the same time, AI moves very fast and it didn’t make sense to compromise by restricting yourself to one catch-all platform that couldn’t leverage best of breed tools.
Dev to Prod: What worked in the development environment (ex. local laptop) always broke in the production environment (ex. cloud cluster). This involved inefficient handoffs between teams and often required ML practitioners to learn to work with complicated and unfamiliar tooling and infrastructure (Kubernetes, Terraform, etc.). All of this increased the maintenance challenge (ex. monitoring) and slowed iteration speed.
So instead of creating yet another ML tooling company, I decided to join a team that has been addressing these precise obstacles with some of the best ML teams in the world and has an even bigger vision I could stand behind. Made With ML allowed me to learn about ML across many industries I’d previously thought I’d personally never be able to impact. I had the opportunity to help many teams implement/improve their ML platforms to make it easier and more reliable to deliver value with ML. And now I’m beyond excited to continue and accelerate this mission with the Anyscale team!
Joining the Anyscale team doesn’t mean compromising on Made With ML’s original purpose — an objective platform where the community can learn to responsibly deliver value with ML. And we’ll continue to push the quality bar on how to design, develop, deploy and iterate on production ML applications. To see this in action, check out the new experience we launched on Made With ML that addresses (hands-on) all the obstacles I mentioned above.
Want to learn more about Ray and how leaders in industry are leveraging it for their ML efforts? Join us at the Ray Summit. |